Digital advertising is a dynamic world where the necessity for privacy and data security is escalating rapidly. Consumers, publishers, and regulatory bodies alike are advocating for improved privacy standards.
At the same time, users want to receive a personalized advertising experience. This means that AdTech companies must strategically incorporate privacy-enhancing technologies (PETs) to protect user data, maintain their privacy, and deliver targeted ads.
In this blog post, we’ll explore the possibilities that PETs offer for enhancing user privacy.
Key Points
- Privacy-enhancing technologies (PETs) are critical for improving data protection in the AdTech industry by balancing between data privacy and the delivery of a personalized advertising experience. PETs primarily focus on minimizing the collection and use of personal data, as well as the amount of data processed, while maximizing data security to protect consumer privacy.
- Most AdTech platforms can implement a variety of PETs that include differential privacy, secure multi-party computation, techniques for anonymizing personally identifiable information, and solutions that incorporate PETs for secure data sharing (such as data clean rooms).
- Each AdTech platform can leverage different PETs to enhance user privacy.
- AdTech companies like ad networks, DSPs, SSPs, and ad exchanges can utilize PETs such as differential privacy tokenization, homomorphic encryption, secure multi-party computation, federated learning, and pseudonymization.
Please note: For the purposes of this article, we will be using the term privacy-enhancing technologies (PETs) to represent all technologies, techniques, and strategies that improve the quality of data protection.
What Are Privacy-Enhancing Technologies
Deloitte defines privacy-enhancing technologies (PETs) as a broad spectrum of “data privacy protection approaches, from organizational to technological.” PETs seamlessly integrate elements of cryptography, hardware, and statistical methodologies to guard against unauthorized processing or sharing of consumer data. They act as protective measures, ensuring the secure handling of sensitive information.
PETs help ensure data is secure by focusing on three key pillars:
- Minimizing the collection and use of personal data
- Maximizing data security to protect consumer privacy
- Minimizing the amount of data processed
We described most AdTech-related PETs in our previous posts: What are Privacy-Enhancing Technologies (PET) in AdTech and The Benefits of Privacy-Enhancing Technologies (PETs) In AdTech.
We Can Help You Build an AdTech Platform
Our AdTech development teams can work with you to design, build, and maintain a custom-built AdTech platform for any programmatic advertising channel.
The Key Privacy-Enhancing Technologies (PETs) that AdTech Companies Can Utilize
Most AdTech platforms can implement technologies that include differential privacy, multi-party computation, techniques for anonymizing personally identifiable information (PII), and solutions that incorporate PETs for secure data sharing (e.g., data clean rooms). Each piece of technology helps to achieve a different goal, resulting in enhanced data protection for users.
Differential Privacy: Adding Noise to Collected Data
Differential privacy (DP) provides a framework for sharing information about a dataset without revealing specifics about individuals. Differential privacy (DP) techniques introduce statistical noise into the data collected by publishers and advertisers, ensuring that users’ identities remain anonymous while still enabling valuable insights to be derived from aggregated data.
To add some clarity, differential privacy is achieved by incorporating a certain level of randomness into an analysis.
Unlike conventional statistical analyses that involve calculating averages, medians, and linear regression equations, analyses conducted with differential privacy introduce random noise during computation.
The “random noise” elements refer to randomized perturbations or statistical variations introduced into data calculations or results, typically through algorithms or mechanisms such as Laplace noise or Gaussian noise.
As a result, the outcome of a differentially private analysis is not precise but an approximation, and if the analysis is performed multiple times, it may produce different results each time.
Examples of applying DP in the AdTech landscape include:
- APIs created for the Google Privacy Sandbox initiative, which prevent the Chrome browser from sharing identifiable data until it can be combined with many other users’ data points.
- Apple’s implemented differential privacy in its systems, starting with iOS 10. When users choose to share Diagnostic and Usage Data, Apple applies the principles of differential privacy to safeguard that data. It further applies DP to Web Browsing data.
- Facebook used differential privacy to protect a trove of data it made available to researchers who were analyzing the effect that sharing misinformation has on elections.
Secure Multi-Party Computation (MPC): Safe Data Computing
Secure multiparty computation (MPC) allows two or more parties to perform computations on their collective data without revealing their individual inputs. However, the mathematical protocols of MPC do not attempt to hide the identities of the participants; this can be achieved by adding an anonymous-communication protocol.
MPC enhances privacy, as parties can gain insights from the combined data set without exposing their private information.
Examples of MPC in the AdTech landscape include:
- Magnite creates synthetic stable IDs using encrypted data to activate the data without accessing the raw datasets.
- DOVEKEY by Google simplifies the bidding and auction aspects of TURTLEDOVE by introducing a third-party KEY-value server.
- The Interoperable Private Attribution (IPA) initiative by Mozilla and Meta aims to provide a framework for attribution measurement without tracking users.
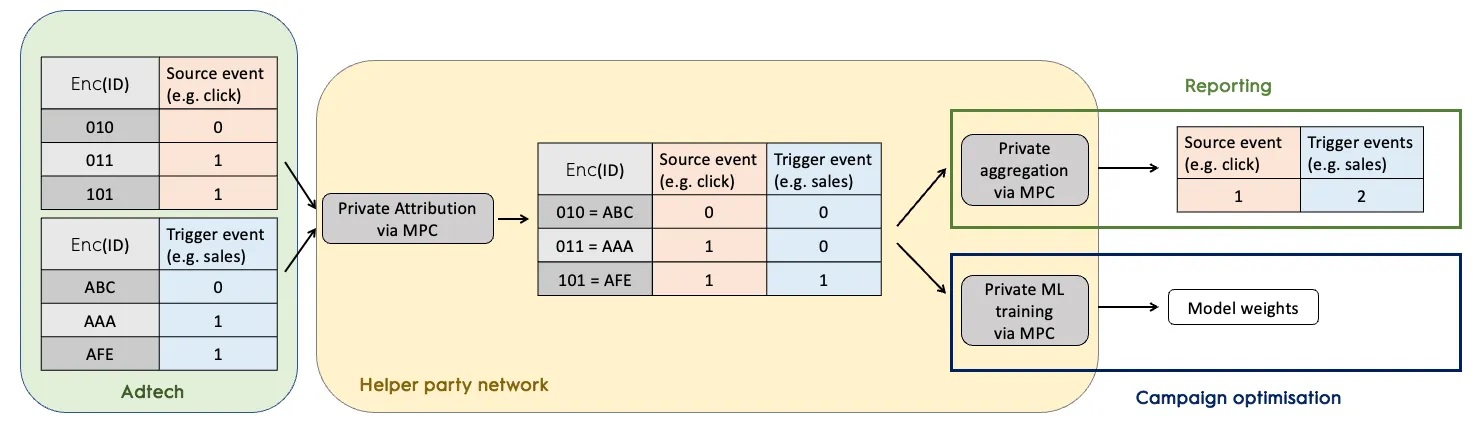
Anonymization, Pseudonymization, Encryption, and Tokenization Techniques: Replacing Personally Identifiable Information (PII)
Anonymization, pseudonymization, encryption, and tokenization are techniques for replacing PII with non-sensitive information tokens. These tokens are created to “cover” raw data so it isn’t exposed.
The names of the techniques may suggest similarities or differences between them. However, in the context of the AdTech industry, it is important to know the differences.
Anonymization
Anonymization involves the process of transforming data in such a way that it no longer identifies or can be linked to an individual. The goal is to remove any identifying information entirely, making it practically impossible to re-identify specific individuals from the data.
Anonymization techniques applied in the AdTech industry can include aggregation, data masking, and other methods that significantly reduce the risk of re-identification.
Anonymization is often used by AdTech platforms to:
- Perform statistical analysis
- Conduct basic audience segmentation
- Generate insights
Encryption
Encryption is a security measure that involves transforming data into a coded form — often referred to as ciphertext — that cannot be understood by anyone who doesn’t have the key to decode it.
In the context of AdTech, encryption can be used to secure PII when it is being transmitted between systems or when it’s stored in databases. The encryption ensures that even if the data is intercepted or accessed without authorization, it will remain unreadable and, therefore, useless to the attacker.
Encryption is often used by AdTech platforms to:
- Secure data transmission
- Store data securely
- Protect user privacy
- Comply with data protection regulations
Pseudonymization
Pseudonymization involves replacing or modifying personally identifiable information (PII) with pseudonyms or aliases. The original data is transformed in a way that makes it more challenging to identify individuals directly but still allows for certain types of analysis or processing to be performed.
Pseudonymized data retains the potential for re-identification if the pseudonyms are somehow associated with the original identities.
Pseudonymization is often used by AdTech platforms to:
- Deploy targeted advertising
- Measure campaign effectiveness
Tokenization
Tokenization is a method of substituting sensitive data with unique tokens that have no meaning or value on their own. The technique allows for efficient data processing and storage without revealing actual personal information.
AdTech platforms may tokenize PII, such as email addresses or device identifiers, by replacing them with randomized tokens.
Tokenization is often used by AdTech platforms to perform:
- Deploy targeted advertising
- Track users
- Measure campaign effectiveness
Data Clean Rooms (DCR): Data Sharing, Targeting, and Measurement
Data Clean Rooms (DCR) are controlled environments that allow multiple processes to be applied to data to protect it. The main purpose of DCRs is sharing and analyzing data without exposing the raw information in order to provide insights while simultaneously safeguarding user privacy.
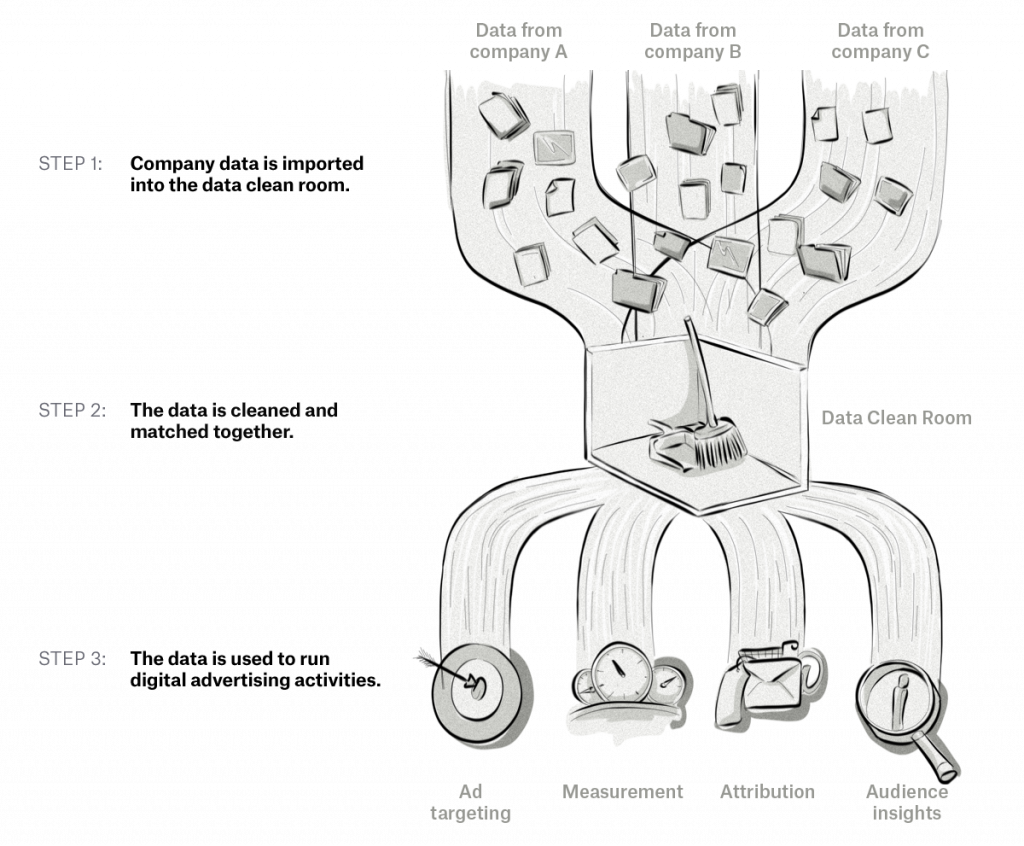
Currently, the AdTech industry has two main types of DCRs.
The first type is represented by AdTech walled gardens — i.e., Google, Amazon, and Facebook — each of which runs media clean rooms from which they deliver hashed and aggregated data to companies that use their advertising platforms.
The second type is represented by independent AdTech companies, such as LiveRamp, Snowflake, Aquilliz, and Decentriq, that provide companies with ready-to-use data clean rooms to use across different industries and digital advertising channels.
In our interviews with Juan Baron, Director of Business Development & Strategy (media & adv) at Decentriq, and Gowthaman Ragothaman, CEO of Aqilliz, we learned that in the digital advertising space, the most common use cases of DCRs are:
- Media planning
- Retargeting
- Creating audience segments
- Activation
- Measurement
- Providing predictive analytics
- Attribution
Privacy-Enhancing Technologies for AdTech Companies
As mentioned before, the integration of privacy-enhancing technologies within AdTech platforms is a crucial component for ensuring user data protection and adhering to evolving global data privacy regulations. Another benefit of adopting these technologies is providing a competitive edge in a privacy-conscious market.
PETs for Ad Networks
Ad networks have several privacy-enhancing technologies at their disposal to ensure user data protection.
By using tokenization and differential privacy, ad networks can deliver effective, targeted advertisements while also respecting and preserving user privacy.
Contextual advertising reduces the need for personal data collection by displaying ads on matching websites. Differential privacy prevents the identification of individuals while analyzing reports. And, tokenization replaces sensitive data — e.g., e-mail addresses — with non-sensitive tokens, securing data in case of data breaches and identity theft attempts.
PETs for Demand-Side Platforms (DSPs)
By incorporating data clean rooms, homomorphic encryption, differential privacy, and secure multi-party computation, DSPs can navigate the balance between ad personalization and user privacy.
Data platforms that incorporate PETs, such as data clean rooms, can provide secure environments for data processing and analysis, ensuring that sensitive user information remains protected.
Homomorphic encryption allows DSPs to perform computations on encrypted data without decrypting it, thereby securing data while still making it usable for ad targeting.
Similar to ad networks, DSPs can also leverage differential privacy, introducing statistical ‘noise’ into data to prevent the identification of individuals while still allowing meaningful analysis for ad targeting.
Secure multi-party computation enables data insights from multiple sources without exposing raw data, further enhancing privacy.
PETs for Supply-Side Platforms (SSPs)
Supply-side platforms (SSPs) can also leverage various privacy-enhancing technologies to ensure the safety of user data while optimizing ad space for publishers. By adopting differential privacy, federated learning, and homomorphic encryption, SSPs can effectively protect user data while optimizing ad placements.
To aggregate and analyze user data (e.g., analyze trends and behavior) without infringing on user privacy, SSPs can leverage differential privacy. This technique introduces statistical ‘noise’ into data, thereby safeguarding individual identities.
Federated learning, an advanced machine learning algorithm that enables data analysis and processing on the device it was collected on, can strengthen ad optimization by building more accurate models of serving ads.
Homomorphic encryption can protect user data while enabling SSPs to build encrypted user profiles. These encrypted profiles can be used to target ads effectively while the underlying user data remains secure and private.
PETs for Data Platforms (DMPs)
Data platforms, including data management platforms (DMPs), customer data platforms (CDPs), and data clean rooms, are central hubs for collecting, integrating, and managing large amounts of structured and unstructured data from different sources. Because of their primary function, they need to maintain user privacy on a high level.
Both differential privacy and pseudonymization can enhance the process of audience segmentation and data sharing in DMPs. DMPs can use these techniques to create anonymized or pseudonymized user segments, enabling precise ad targeting without compromising individual user privacy.
PETs for Ad Exchanges
Ad exchanges are digital marketplaces for buying and selling ad inventory from multiple DSPs and SSPs where prices are determined through real-time bidding (RTB) auctions.
Incorporating differential privacy and secure multi-party computation can help protect the sensitive information of users during the bidding process. By adding statistical noise to the data used for bidding, ad exchanges can ensure that bidding does not result in the leakage of sensitive user information.
Other use cases for differential privacy include reports and analytics modules.
By using differential privacy, data and insights can be displayed in a way that prevents the identification of individual users, thereby enhancing privacy.
Ad exchanges can also utilize secure multi-party computation to match advertisers and publishers based on their respective criteria, without revealing the private information of either party.
PETs for Ad Servers
Ad servers store and deliver ads to websites and apps and provide reports on ad performance. With the technologies and techniques of differential privacy, encryption, and federated learning, ad servers can enhance user privacy significantly.
Differential privacy can ensure that the processes of data analysis do not expose sensitive user information.
Encryption in ad servers secures user data by encoding it into a format that can only be accessed with the correct decryption key.
For ad servers, federated learning allows for data analysis without needing to share the data itself, enhancing user privacy.
Summary
The adoption of privacy-enhancing technologies in the AdTech industry is an important step toward respecting user privacy and ensuring data security. With the correct application of these technologies, platforms can deliver effective advertising while also prioritizing the privacy and security of their users’ data.
We Can Help You Build an AdTech Platform
Our AdTech development teams can work with you to design, build, and maintain a custom-built AdTech platform for any programmatic advertising channel.