Content personalization is a method of making the website experience equally relevant for everyone visiting. This basically involves tailoring the website’s content to accommodate each user’s preferences.
On top of that, good personalization platforms also perform cross-device tracking, creating a seamless experience across all channels, including web, mobile, and email. Then, with every successive visit, the front page of the store can be dynamically altered to display only the relevant content, such as personalized recommendations and promotions.
What is the Purpose of Content Personalization?
Personalization is broadly employed to meet a variety of goals, depending on the business model. For example, streaming media providers like Netflix or Spotify meticulously craft their recommendation systems for higher satisfaction of their audience, without directly benefiting from it in any way. In e-commerce, the purpose of content personalization is to help visitors find the desired products more quickly and make them convert. Google uses search personalization to show different results to different people, signed in or otherwise. Facebook prioritizes the posts every user might prefer to see.
Personalization of content can be achieved with a number of methods, depending on factors like the amount of user data at hand, scale, and privacy concerns.
Machine-Learning Personalization
Machine-learning (or predictive) personalization allows for easier scalability, as it does not require the manual creation of the rules for each segment. It better lends itself to product or content recommendations with a number of smart tactics to recycle and repurpose existing content. Its practical applications range from video- and music-streaming services, retailers and travel agents or airlines, and other services with vast, dynamically changing offers.
Predictive content personalization involves real-time segmentation of users and employs machine-learning methods to automatically match each person to a group of like-minded users. Then, the recommendations are based on the actual actions (like purchases) of the people in the segment, making it incredibly accurate. Predictive personalization also analyzes visitor behavior on different pages and predicts possible purchase intent before a single dollar is spent, shortening the path to conversion.
Rules-Based Content Personalization
With rules-based content personalization, the content is generated and targeted based on a number of manually predefined rules. The content is modified and displayed to visitors based on information such as demographics, location, and past visits to the site.
This method is better suited to smaller sites that don’t necessarily have vast amounts of user data at their disposal, and where the predictive personalization method would be of no use or simply overkill.
The Differences
The predictive and rules-based personalization methods both aim for the same goal, but differ in a few key areas.
Carved in Stone vs. Dynamic
Rules-based personalization efforts involve the creation of a set of rules that display specific content to different visitors, but this method is not dynamic—each version of the landing page has to be manually designed. Then, each rule works consistently, displaying the same result until someone changes it. The creation of rules on variations of the website is a manual process.
Conversely, predictive personalization is dynamic. It taps into the potential of machine learning and once set, does not require human interaction. It uses algorithms that try to make sense of every visitor’s behavior and personalize the site accordingly.
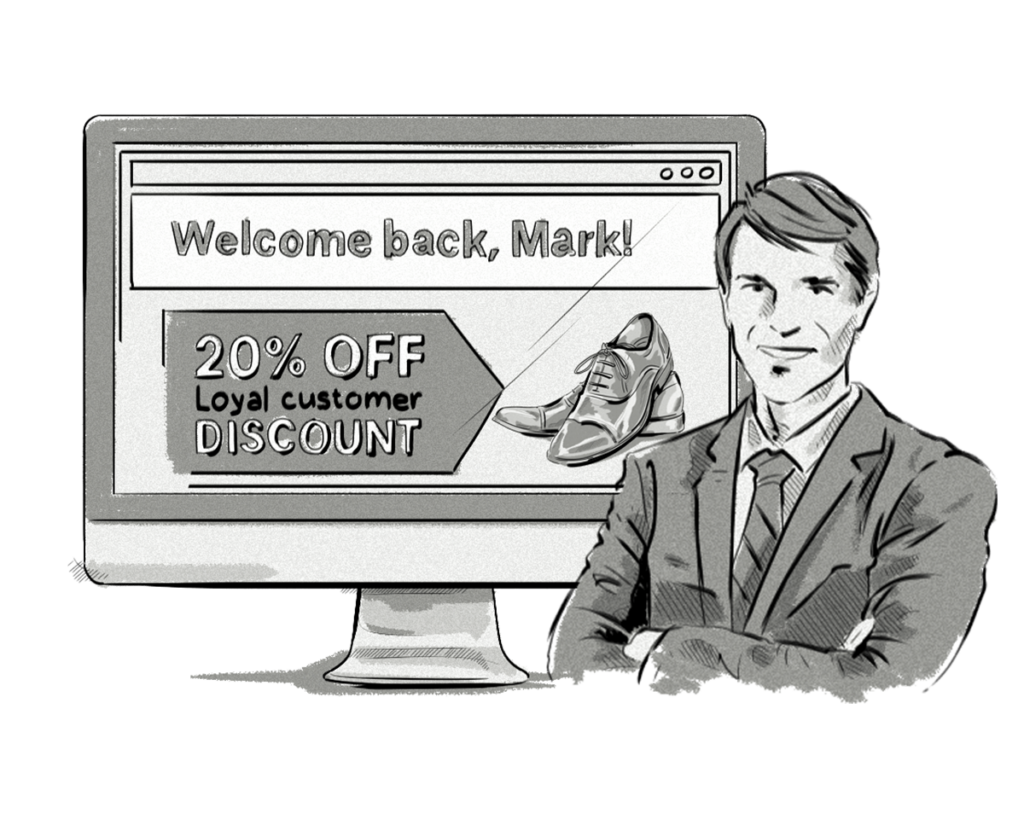
Suitability for E-commerce
Both rules-based and predictive personalization are good for e-commerce, but have slightly different applications.
Rules-based personalization works well even with little easily accessible data at hand. It can be used to display personalized callouts (using each user’s name, for instance), or pop-up messages to new customers. For example, a mobile-phone store can display mobile-phone banners only to known visitors who have expressed interest in a specific brand and, say, offer them applicable discounts and promotions. In this way, first-time, return, and high-value visitors can be addressed differently.
The predictive personalization method is more complex, as it can offer visitors specific products (“often bought together,” “you may also like…,” “people like you also bought…” etc.) based on their demonstrated behavior. Because it uses machine-learning algorithms, no manual work is required to put these rules into place. This is specifically important for websites with a big product offer, and the method pays off if there are a lot of visitors to the site.
The content optimized with predictive personalization can be used in search box recommendations, results, email messaging, product lists, checkout pages, etc.
Types of Data Used
Rules-based personalization heavily relies on explicit information like demographic and contextual data, or anything else the visitors to the site have willingly provided.
Such data is collected by tracking the UTM codes in the visitor’s URL, their browser data, and on-site and in-product analytics platforms.
Predictive content personalization, on top of explicit demographic data, also taps into implicit data. For example, a rule can be implemented to identify a returning user, but predictive personalization will provide the recommendations for the segment. The tailored content is prepared based not only on the explicit information and behavior of the user, but on the intent as well.
Which Method is Better?
It’s hard to definitively say one method is better than the other, as they serve slightly different purposes. The rules-based method is more basic and should suffice for 90% of applications. It is also the ideal starting point for all personalization efforts, and should always be implemented before a foray into predictive is made. In this way, you will be able to find out if the transition from rules-based to predictive is needed in the first place.
When a system utilizing rules-based personalization grows, there is more and more data to tap into, but there are also more and more exceptions to handle. Naturally, there will be rules to handle all these exceptions. In the long run, however, such a system is bound to get out of hand; the data will eventually start changing faster than the rules used to manage it. A number of false positives and negatives can trigger particular rules with no useful actionable results.
This problem is inherent to all traditional, “manual” and rules-based personalization—there is always a point, where the number of rules and exceptions becomes unwieldy. This challenge can be overcome by machine-learning methods, which are better suited to handle the volume, velocity, complexity, and variety of data. Even more so, the volume and velocity of data makes the machine-learning systems stronger.
Is the Predictive System the More Future-Proof Solution?
Very simply put, convenience, scalability, and low operational cost make the predictive personalization efforts more future-proof. This is true especially when dealing with a blend of unstructured, structured, and semi-structured data concurrently. Machine-learning systems, in this case, remove the tedious manual task of classifying and tweaking the rules each time.
Summary
Predictive personalization is certainly a potent method to craft a user-centered experience, but your website simply may not need it that badly. Also, although there are specific differences between rules-based and predictive personalization, there is no reason they couldn’t coexist within one site. For example, rules-based personalization can be used to identify whether the visitor is a returning or new user (based on explicit data), while predictive personalization can simultaneously be used to display recommendations (implicitly, based on behavior) – all with the ultimate goal of streamlining the user experience.